2.1 Common approaches for revenue forecasting
Revenue forecasts provide critical context to governments to inform their decision-making on budgeting and revenue policy. However, whether we should use simple or sophisticated methods remains an outstanding question. Favero and Marcellino (2005) conducted a comprehensive comparison of different forecasting methods used by governments to predict fiscal variables, including univariate autoregressive and moving average models, vector autoregressions (VARs), and small-scale semi-structural models. They find that simple univariate time series methods tend to provide effective and unbiased forecasts, outperforming multivariate models that rely on a system of macroeconomic variables. They attribute this to the difficulty of modelling the joint behaviour of multiple macroeconomic variables in a short sample with significant institutional and economic changes, as well as the robustness of simple methods in accounting for structural breaks.
Conversely, some more recent studies show that multivariate models can improve forecast accuracy. These studies usually utilise longer sample periods and more advanced forecasting techniques, compared to Favero and Marcellino (2005). For instance, Carriero, Mumtax, and Theophilopoulou (2015) present evidence that their Bayesian Vector Autoregressions (BVARs) are more effective than simple forecast approaches, as they can better summarise the information contained in a large dataset and allow for time variation in the coefficients. The authors demonstrate that BVARs outperform simple models in both point and density forecasting using data from the United States and Europe. In our study, we add to this ongoing discussion regarding the relative performance of different revenue forecasting techniques by incorporating machine learning models to predict taxation revenue in Australia.
Machine learning methods have been used for forecasting as early as Hu’s (1964) work on weather forecasting. With technological advancements, there has been a substantial increase in the number of studies utilising machine learning for time series forecasting. However, evidence of the effectiveness of these algorithms remains inconclusive. On one hand, some recent studies show that machine learning methods can outperform traditional methods in forecasting stock market returns (Gu, Kelly, and Xiu 2020), predicting inflation (Medeiros, Vasconcelos, Veiga, and Zilberman 2021), and nowcasting GDP growth (Babii, Ghysels, and Striaukas 2022). On the other hand, other studies suggest that machine learning algorithms do not outperform simple models such as ARIMA (Makridakis, Spiliotis, and Assimakopoulous 2018) and some are not even better than random walks (De Gooijer and Hyndman 2006). Thus, our paper is a case study that also contributes to the emerging literature on the effectiveness of machine learning algorithms in time series forecasting.
2.2 Taxation revenue in Victoria
The Victorian Government collects revenue from a range of taxes, including payroll tax, land transfer duty, land tax, gambling taxes, motor vehicle taxes, and insurance taxes.1 Taxation revenue is utilised to fund public services and infrastructure. Every year, Budget Paper No. 5 Statement of Finances contains the Estimated Financial Statements and accompanying explanatory notes that set out the forecast financial results for the Victorian general government sector for the next four years.
This study will focus on forecasting the two biggest tax lines of the state, namely payroll tax and land transfer duty. Victoria imposes payroll tax on employers when the total taxable wages exceed a specific threshold.2 Land transfer duty, on the other hand, is one-time tax levied on most real estate transactions and is calculated based on dutiable value of the property.3 In addition to their importance, we are focusing on these two taxes because they are closely tied to the employment and housing markets, and previous research suggests that machine learning methods can effectively predict their trends (e.g. Gogas et al. 2022; Kreiner and Duca 2020; Milunovich 2020).
It is worth noting that forecasting land transfer duty and forecasting payroll tax present different issues. As discussed in DTF’s 2023 submission to the Victorian Parliamentary Inquiry on land transfer duty fees, forecasting land transfer duty is typically considered a more challenging task (DTF, 2023):
'Revenue forecasts provide critical context to governments to inform their decision-making on budgeting and revenue policy. While having accurate forecasts for all revenue sources is important, land transfer duty typically contributes around 9 per cent of total general government sector revenue each year, and its cyclical volatility can disproportionately affect overall revenue forecast errors when compared to most other sources of taxation revenue.'
2.3 Land transfer duty and payroll tax revenue from 1990 to 2022
Figures 1a and 1b show the quarterly payroll tax and land transfer duty revenue (seasonally adjusted) from 1990 to 2022. We can see that while both series show an upward trend, land transfer duty revenue is much more volatile than payroll tax revenue. This aligns with our expectation, as land transfer duty is influenced by movements in the housing market, which is typically more volatile than the labour market that affects payroll tax revenue. In YR2021-22, payroll tax and land transfer duty generated around $1.7 billion and $2.6 billion for the Victorian Government per quarter, respectively.
We can also see the significant shifts in the patterns of payroll tax revenue and land transfer duty revenue since 2020. Specifically, payroll tax revenue experienced a steep decline at the onset of the COVID-19 pandemic due to the weakened labour market and government payroll tax relief policies, while land transfer duty revenue has seen a substantial increase since late 2020, in correlation with the robust growth in property prices.
Figure 1a. Payroll tax from 1990-2022
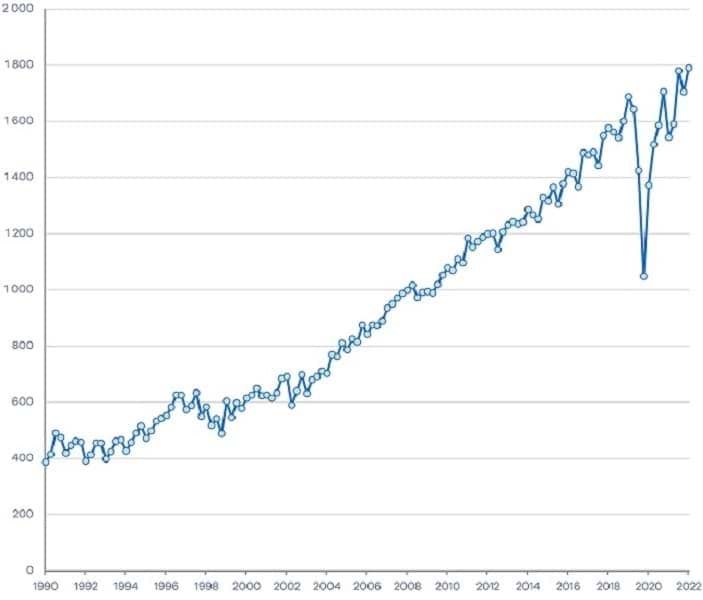
Figure 1b. Land transfer duty from 1990-2022
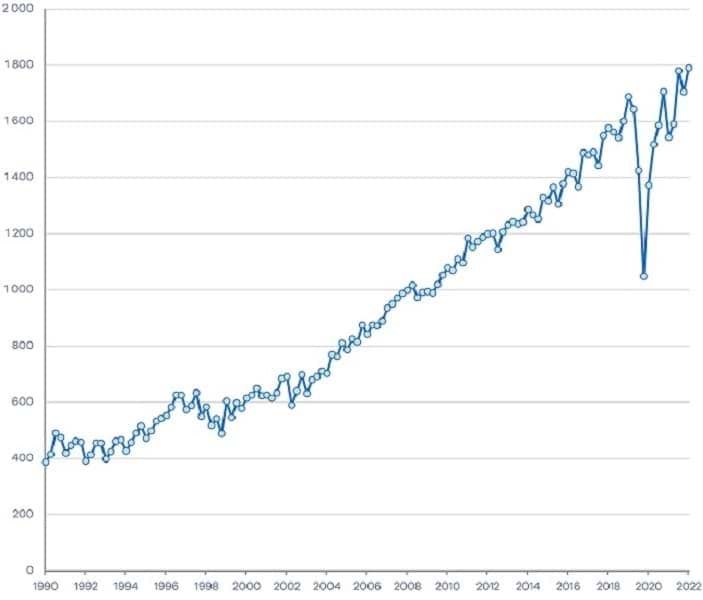
Footnotes
[1] In 2022-23, the total taxation revenue of the Victorian Government was about $32.4 billion. View the 2022-23 Financial Report.
[2] For more information on payroll tax, visit the State Revenue Office website.
[3] For more information on land transfer duty, visit the State Revenue Office website.
Updated