- Published by:
- Department of Treasury and Finance
- Date:
- 1 Oct 2023
Rebecca Valenzuela1, John Inekwe2 and Hnin Inioluwa Tun Agbaje3.
1. Department of Treasury and Finance, Victoria
2. Monash University
3. University of Adelaide
Author contact details: veb@dtf.vic.gov.au.
Disclaimer: The views expressed in this paper are those of the authors and do not necessarily reflect the views of the Victorian Department of Treasury and Finance (DTF).
Suggested Citation: Rebecca Valenzuela, John Inekwe and Hnin Inioluwa Tun Agbaje (2023), A region-based examination of electric vehicle take-up rates in Australia. Victoria’s Economic Bulletin, October 2023, vol 7, no 1. DTF.
Abstract
The study examines electric vehicle (EV) adoption rates across regions in Australia by utilising a nonlinear factor framework based on club convergence analysis. The results reveal the existence of four distinct regional groups (called convergence clubs) in terms of EV take up rates, and that this grouping has been fairly stable between 2013 and 2020.
The study also finds that regions incorporating city centres are more likely to cluster as top adopters than peripheral regions. About 50 per cent of the regions in the study appear in the higher-formed group, which signifies an improvement in environmental outcomes. The paper models determinants of EV adoption using region characteristics and finds that higher levels of green awareness, per capita income, and higher levels of education of residents within a region are significant positive influences. There is also some evidence pointing to the positive influence female populations exert on EV adoption and that higher within-region income inequality leads to lower EV adoption.
1. Introduction
Global indicators show that Australia has been notably slow in tackling transport emissions.
Global statistics show that Australia’s per capita transport emissions are 45 per cent higher than the OECD average1. Given its geography and land size, the country’s transport industry is a large and an important contributor to the economy's growth and development. By the same token, the transport industry is the country's third largest source of greenhouse gas emissions, and as such it is a significant source of climate change risk for residents in the long term.
Given the Australian governments’ commitments to net zero emissions by 2050, led by Victoria’s steadfast resolve to cut the state’s emissions by 75-80 per cent by 2035 (Victoria’s Climate Change Act 2017), gaining a clear understanding of factors that influence demand and take-up rates of electric vehicles is a move in the right direction. According to the latest report by the Australia Climate Council, to reach the Australian government’s target of net zero carbon dioxide emissions by 2035, 75 per cent of Australia's new car sales by 2030 need to be electric (see Purtill, 2021). The last 12 months appear to have been a good year towards this end with the Electric Vehicle Council (EVC) reporting rapidly rising EV sales. Accordingly, 46,624 EVs were sold in Australia between Jan and June 2023 which is almost 3 times higher than the same period in 2022. EVC further reports that EVs this year represent 8.4 per cent of all new cars sold in Australia – a rate which is more than doubled the 3.4 per cent observed last year2.
While the country’s EV market continues to expand, Australia’s EV sales share of 8.4 per cent is still a long way behind reported figures in Europe: 88 per cent in Norway, 54 per cent in Sweden, 35 per cent in Netherlands, 31 per cent in Germany, 23 per cent in the United Kingdom and 21 per cent in France (IEA 2023). The strong performance of the domestic EV market, however, meant that Australia’s EV sales share in 2022-23 has caught up with that of the United States, where the EV sales rate has been slow to improve relative to Europe. A 2021 survey by The Australia Institute found that two-thirds of Australians support all new car sales to be zero emissions vehicles by 2035 (The Australia Institute 2022), while EVC’s Consumer Attitudes Survey in 2021 found that about half of respondents saw themselves driving an electric car in 2030 (EVC 2021). However, the same reports also show that domestic consumer willingness to buy an electric vehicle is generally low, and that regional Australians are more cautious about making 2035 an all-electric vehicle zone compared to their counterparts in more urbanised areas.
It has been noted that rural and regional Australia faced the challenges of distance and limited access to EV charging, but the authors believe there is more to it than that. Australia is a very large continent with a wide variety of landscapes and climates – including deserts in the centre, rich tropical rainforests in the north-east, and lush mountain ranges in the south-east. Its mixed-market economy is richly endowed with natural resources which are unevenly spread across the land. The economic profile of states, territories and regions – and the consumers therein – vary widely, with some having greater level of dependence on primary resources and others mainly drawing income from the service and financial industry. The diversity of the national economy is also manifested in its energy consumption. Coal and natural gas remain the main source of energy for the country, accounting for 64 per cent of all energy in 2022, while energy from renewable sources such as wind, solar, hydrogen and bioenergy accounted for 35.9 per cent3 of the country’s total electricity generation (CECA 2023). Given this, it is apparent that Australia’s regions can differ in their environmental goals.
In this study, we explore the observed variations in regional adoption rates to understand preferences and how they can affect EV adoption behaviour over the foreseeable future. We utilise club convergence analysis to understand the underlying patterns of EV adoption across the country, and gain insights into factors driving the direction and speed of EV adoption in the medium term. Additionally, we develop models of consumer preferences which can provide valuable clues into the role of green awareness on the speed of EV adoption, amongst other more well-known factors. To our knowledge, the potential impact of green awareness on EV adoption has not yet been empirically tested, so this study will allow us to understand how economic, social, technical and gender status across regions can affect the evolution of Australia’s EVs usage.
The remainder of the paper is structured as follows. In Section 2, we review the literature on EV adoption to focus on factors that influence EV adoption decisions, examine lagging EV adoption regions and look at various modelling methods used in previous work. In Section 3, we introduce the club convergence methodology and describe in detail how we apply it to the case of EV adoption. We discuss the dataset and our key variables in Section 4. In Sections 5 and 6 we present our empirical results with discussion and analysis. Section 7 concludes.
Footnotes
[1] Climate Council, Transport emissions: Driving down car pollution in cities(opens in a new window), accessed 27 February 2023.
[2] Electric Vehicle Council, Australian Electric Vehicle Industry Recap 2023(opens in a new window), accessed 10 October 2023.
[3] This is up from 32.5 per cent in 2021.
2. Literature review
EV adoption is an area of research that is very popular and is continuing to grow.
There is a wealth of recent works that have focused on determinants alone. In this review, we will discuss key factors that have been shown to impact on EV adoption rates. We will also review studies that have focused on lagging regions in terms of their EV adoption.
As with any purchase, consumers often make decisions by considering the dollar price of the item against the expected benefits that can be derived from the product. As cars become an increasingly pricier commodity, there is greater care devoted to these net value assessments. It is clear from the literature that EVs are highly desired as they contribute to a cleaner environment, typically have no congestion charges, operate at lower running costs, benefit from targeted government funding and generally offer a better driving experience (Mustafa et al. 2021; Xiong et al. 2020; Zhang et al. 2021). In the early days of the electric vehicle market, it is generally known that potential consumers were deterred by the EVs high purchase price and lack of charging infrastructure that can support the need for frequent battery charging. However, EV prices have since come down due to lower-production costs, more competition on the supply side and significantly improved charging infrastructure.
Given Kyoto protocol commitments, governments of many industrialised countries have designed subsidy and incentive packages to lower the net cost of acquiring an EV and make adoption a more attractive proposition. Unfortunately, these initiatives tend to be offset by other market-based influences which also have a strong impact on the net price or value of an EV. For example, early studies of Diamond (2009) and Beresteanu and Li (2011) show that petrol prices are a more significant influencing factor in the adoption of hybrid EVs, compared to government incentives. In more recent times, when EV prices have reduced significantly, the increased availability of charging infrastructure has become a major factor in favour of adoption (Salisbury and Toor 2016; Mersky et al. 2016; Hardman et al. 2017).
On the role of consumer characteristics, Potoglou and Kanaroglou (2007) found that in Canada, younger people and those with a university degree are more likely to adopt alternative-fuelled vehicles, and that the demand for high-energy consuming vehicles, such as vans or sport utility vehicles (SUVs), diminished if respondents lived in dense and diversified urban areas. Relatedly, Hidrue et al. (2011) found that being younger, having a higher education (bachelor's degree or above), and higher levels of green awareness increased consumer orientation towards EVs, while income and being a multi-car household did not have a significant impact on being in the EV class. These results are later corroborated in Ritter et al. (2015) which showed that socioeconomic status, such as income and educational level, played a significant role in influencing the consumption of green products generally.
On the role of attitude and preferences in EV adoption, there is growing evidence in the literature showing that the influence of these factors is not just significant but also dynamic. For example, Mau et al. (2008) demonstrated that consumer preferences in choosing between conventional and new technologies can change with market conditions, and that the importance consumers place on certain attributes of a new technology, including that of an electric vehicle, also changes as it gains market share. One study concluded that the environmental aspects are less important for consumers than anticipated, despite the concerns about climate change and renewable energy transition (Anastasiadou and Gavanas 2022). There is also new literature analysing the use of EVs as tools for enhancement of self-image and reputation (Li et al. 2022; Buhmann and Criado 2023).
From a modelling perspective, the two most common approaches to understanding consumer preferences for electric vehicles are discrete choice models or latent class models. Hidrue et al. (2011), Mohamed et al. (2016), Ferguson et al. (2018) and others have pointed out that latent class models are preferable compared to other discrete choice methods, because respondents can be grouped into a range of preference classes based on their attitudes and socioeconomic characteristics. There was a notable scarcity of studies about regions lagging in EV adoption, however – we found only one study that has investigated regional factors. Abotalebi et al. (2019) analysed the low adoption rates in Atlantic Canada by comparing EV outcomes to leading English ‑speaking Canadian provinces, namely British Columbia and Ontario. Using data from a household survey and a latent class random utility model, the study finds that EV driving distance range, maintenance cost, free parking, and access to high occupancy vehicle lanes are not significant attributes in the Atlantic model. With respect to segmentation, the adoption of EVs in the Atlantic model increases with youth, education, and progressive attitudes towards the environment, while income is not a determining factor.
In Australia, publicly available data from the Australia Bureau of Statistics (ABS)4 and the Bureau of Infrastructure and Transport Research Economics (BITRE)5 show that since EVs were introduced nationally, capital cities and coastal cities tend to have a higher proportion of EVs than other regional areas. However, these reports mainly present descriptive tables, and while they present detailed information on EV use and purchase, no systematic modelling was undertaken. This research collects relevant datasets to fill the analytical gap in the understanding of EV adoption rates in Australia. It also provides an opportunity to illustrate the feasibility of the convergence club methodology to analyse trends in this field of study.
Footnotes
[4] Australian Bureau of Statistics, Motor Vehicle Census, Australia, accessed 15 May 2023.
[5] Bureau of Infrastructure, Transport and Research Economics, Australia’s light vehicle fleet - some insights, accessed 15 May 2023.
3. Methodology
The club convergence approach theory and associated statistical tests developed in Phillips and Sul (2007, 2009) has gained wide popularity as an application for assessing economic outcomes.
The advantage of using this technique lies in its ability to incorporate a nonlinear time-varying factor model in its estimation process.1
This paper demonstrates the feasibility of applying this technique to model the transitional dynamics and long‑run behaviour of electric vehicle adoption in Australia. To facilitate this, we follow Phillips and Sul (2007) [PS hereafter] and first decompose EV adoption components into two parts as follows:

where δit is a time varying idiosyncratic element which measures the deviation of each region i from the common trend (µt).
Within this framework, the convergence to a steady state at a future date is anticipated for all N when:

The elimination of the trend component µt and the estimation of δit requires the modification of Eq. (1) such that:

where hit is the relative measure that defines the transition path relative to the average of all regions. An econometric test of convergence can be further formulated to identify a second-round of convergence occurring between clubs. This is achieved by considering a semi-parametric form of δit:

where σit = σi L(t)-1t-α, σi ˃ 0, t ≥ 0, and ξit is an i.i.d (0,1) across i, but weakly time dependent. The function L(t) is increasing in t but diverges as t tends to infinity2. From here, we set the null hypothesis of convergence as H0: δi = δ, α ≥ 0, against the alternative hypothesis HA: δi ≠ δ or α < 0.
The focal point of the technique is the examination of the sign of α. Even if there is a rejection of the null hypothesis of convergence in the panel, the occurrence of potential sub-convergence amongst the clubs cannot be ruled out since there is a likelihood of multiple equilibria.
In validating the null hypothesis, this club convergence technique by PS requires estimation of the following equation:

Where:

represents the square cross-sectional distance-relative transition coefficients.
For the case of b̂ = 2â, a one-sided test of b̂ ≥ 0 against the alternative hypothesis of b̂ < 0 can be constructed as the null hypothesis. At the 5 per cent level, the rejection of the null hypothesis occurs if tb̂< -1.653. The identification of the various clubs is achieved through the robust clustering algorithm proposed by Phillips and Sul (2007).
We now outline below the specific steps we adopted to implement this convergence club technique by PS to examine EV adoption in Australia:
- Order the regions in the panel according to the last observation in the time series. This ranking assumes convergence is more apparent in the most recent observations. If there are significant variations within the region, the average of the last fraction of the sample can be taken to order the panel.
- Select the first k highest regions in the panel to form subgroups Gk for some N > k > 2. After estimating regression (4) for each formed subgroup, select a core group through the following cut-off point criterion: k*=ArgMaxk[tb̂], subject to Mink [tb̂] > -1.65 for k = 2, 3, …, N.
- For the remainder regions and each of the formations, re-estimate equation (4) and add one region at a time to the core group. The inclusion of a particular region in the core group will be determined following this sign criterion [b̂ ≤ 0].
- For the remaining regions, repeat steps 2–3 until there is no further club formation. The procedure is then terminated. When regions in the last group do not have a convergent path, then it can be concluded that they belong to a non-converging group.
As stated by PS, using the sign criterion as the decision rule in step (2) may generate an overestimated number of clubs. They recommend that it is essential to perform a club merging test in addition to estimating equation (4). We employ the Hodrick and Prescott (1997) filter to extract the trend component of the series and to generate the trend that minimises the squared changes in trend and deviations, as follows:

This filter extracts the long-run trends, while eliminating the short-run erratic behaviour of the data4.
Footnotes
[1] Recent examples include Awaworyi et al. (2018a, 2018b); Bhattacharya et al. (2020a, 2020b) and Bhattacharya and Inekwe (2021)
[2] In the PS approach, L(t) = log (t) needs to hold in order to guarantee convergence.
[3] The PS approach requires that the heteroskedastic and autocorrelation consistent standard errors for b are obtained as the ordinary least squares standard errors of the region in equation (4) may be weakly time dependent.
[4] In using the filter, the lambda is set to 10, which is approximately the recommended value for annual data.
4. Data
We obtained information on electric vehicle registrations across 87 regions in Australia, between 2013 and 2020 from the Australian Bureau of Statistics (ABS).
The regions refer to the Statistical Area Level 4 (SA4) administrative regions as defined by the ABS. These regions are spread across the country and their distribution by jurisdiction are indicated in Table 1, column (4). This complete panel includes all eight Australian states and territories, with the region share of each jurisdiction reflecting relative population shares. The primary unit of analysis is the region, and the key variable of interest is the number of electric vehicles that are registered annually1.
As seen, there is a clear difference in EV take-up rates across the states and territories, all of which offer some form of subsidy or tax relief(opens in a new window) for EV buyers. In 2023, NSW led the pack in terms of EV sales, but ACT was the clear leader in terms of the EV share of the vehicle market at 20 per cent. This is due to three complementary factors unique to the ACT:
(i) The ACT had a high concentration of early EV enthusiasts among locals2
(ii) The ACT Government led other governments in being an early fleet buyer of plug-in hybrids and battery EVs
(iii) The ACT Government also offers EV buyers stamp duty waivers, free registration and zero-interest loans at rates that are far above those offered in other jurisdictions.
Table 1. EV market data and distribution of regions across jurisdictions
State/Territory | EV sales (2023) | EV market share (2023) | Number of regions by jurisdictions | Average Regional Growth Rate of EV registrations (2013–2020) |
(1) | (2) | (3) | (4) | |
New South Wales (NSW) | 14,164 | 8.0 | 26 | 36% |
Victoria (VIC) | 11,514 | 7.5 | 18 | 23% |
Queensland (QLD) | 8,683 | 6.8 | 18 | 39% |
South Australia (SA) | 2,106 | 5.6 | 7 | 20% |
Western Australia (WA) | 4,005 | 6.6 | 10 | 15% |
Tasmania (TAS) | 758 | 8.0 | 4 | 17% |
Northern Territory (NT) | 106 | 2.1 | 2 | 2% |
Australian Capital Territory (ACT) | 1,815 | 20.0 | 2 | -23% |
Total | 43,151 | 8.4 | 87 |
Source: Data in columns (1) and (2) are from VFACTS 2023, Federal Chamber of Automotive Industries; numbers in columns (3) and (4) are the authors’ calculations from data gathered from the Australian Bureau of Statistics.
In column 4, we can see the growth rates of EV fleets across the jurisdictions. The strongest growth was observed in QLD and NSW at 39 and 36 per cent annual average respectively. VIC and SA’s EV fleets grew strongly as well at 23 and 20 per cent respectively. The EV fleets of TAS and WA likewise had healthy growth rates of 17 and 15 per cent respectively. Growth rate in the NT was 2 per cent, and -23 per cent in the ACT. The negative growth rate recorded for the ACT is due to a significant drop in numbers between 2019 and 2020, although this has reversed since 2021 (EVC 2022).
Table 2 presents the variables we assembled for each region between 2013 and 2020. We can see that the average number of the vehicle is 216 330, while the average number of electric vehicles across all regions is about 91. This small number clearly indicates the very slow rate of market penetration of EVs compared to non-EVs. To gauge environmental awareness across the regions, we also collected information on the number of small-scale solar panel system installations and total solar water heater installations per year per region. The total number of solar installations was obtained by adding these two series, providing the variable we used to measure the level of green awareness of each region.
Table 2. Summary Statistics of Key Variables, Pooled, 2013-2020
Variable | Mean | S.D. | Min. | Max. |
---|---|---|---|---|
No. of Vehicles | 216,330 | 106,800 | 34,986 | 638,766 |
No. of Electric vehicles | 91 | 199 | 3 | 2,443 |
No. of Solar Installations | 3,079 | 2,444 | 89 | 17,569 |
Total Regional Income ($B) | 10,004 | 6,354 | 841 | 35,761 |
Population Headcount | 285,963 | 158,055 | 37,545 | 883,260 |
- Female population | 144,162 | 79,713 | 18,264 | 439,034 |
- Male population | 141,800 | 78,414 | 19,281 | 444,226 |
Population density (persons/sqkm) | 691.46 | 1,262.96 | 0.10 | 5,689.20 |
Education (% completed Year 12) | 48.52 | 12.871 | 27.3 | 75.5 |
Total Employee Income ($B) | 8,714 | 5,482 | 695 | 30,322 |
Gini Coefficient | 0.466 | 0.042 | 0.389 | 0.61 |
The average regional income was $10 billion, with a large standard deviation indicating a considerable variation across them. On average, the value of regional employment earnings contributed about 87 per cent of total regional income. There are roughly equal numbers of males and females in each region, and on average one in every two individuals has completed Year 12 or higher. The Gini index of inequality across the regions ranges from 0.389 to 0.61, indicating the existence of a medium to high-level inequality all round. Lastly, there appears to be a strong variation in green awareness across the regions as the number of solar installations varied greatly in the sample.
Footnotes
[1] For this study, we will not distinguish between types of EVs; we will leave that for a future exercise when EV data can allow meaningful analysis from finer partitioning of the count data. Additionally, because we are focused on regions and given the data available to use for club convergence analysis, we have avoided needing to classify adopters into more granular kinds eg government, business and private.
[2] See EVC 2022 report for additional details. For a short historical overview, see The Driven, Canberra fast becoming Australia’s little Norway as EV share hits 19 pct.(opens in a new window)
5. Results from club convergence analysis
The analysis of convergence is established upon the premise that the industry structure of each Australian region is influenced by its local natural resources.
It follows that varying administrative regions are likely to differ in their environmental goals and employ different economic strategies to achieve those. In our empirical analysis, we therefore expect regions to shift to a reliance on renewable energy sources at varying speeds.
Table 3 presents the first results of our club convergent analysis using the PS approach. We find that applying the club clustering algorithm to the data has led to the formation of four convergent clubs. The result of testing for full panel convergence among all the regions is also instructive. In the last row of Table 3, we can see that the log (t) statistics value for the entire sample is −46.832, which is less than the critical value of −1.65. The null hypothesis of full panel convergence is thus rejected at the 5 per cent significance level. This means that Australian regions have different transition paths in EV adoption. As this evidence indicates that the regions do not follow a single development path, it becomes conceivable to have a heterogeneous equilibrium with distinct outcomes.
Given that the club clustering algorithm may overestimate the number of clubs, we follow PS and apply a second round of club convergence analysis to the data to test the likelihood of initial clubs merging into larger clubs. This second classification exercise found no other clubs were merged in the process, hence the final number of clubs formed remains at four.
Table 3: Club convergence tests for electric vehicle adoption intensity
Clubs | Beta-coefficient | t-statistic | Standard error |
---|---|---|---|
1 | -0.167 | -1.522 | 0.110 |
2 | 0.165 | 0.883 | 0.187 |
3 | 0.236 | 0.745 | 0.317 |
4 | 1.211 | 3.187 | 0.380 |
All | 1.500 | -46.832 | 0.032 |
Notes: The analysis makes use of the critical value of t(p=0.05) = −1.65 across all cases in testing for the one-sided null hypothesis b ≥ 0 against b<0. Clubs represent the merged adjacent clubs.
In Table 4, we present further results from the club-convergence analysis above. We can see that the first formed club consists of 42 regions from all jurisdictions except for one. NSW is well represented in Club 1 with thirteen of its regions included. VIC has nine of its seventeen regions included while QLD has eight of its eighteen regions included. Regions included in this first formed club are considered the most rapid and active EV adopters in the entire set of regions covered. At 42 out of the 87 regions in Club 1, this group of EV adopters comprise 48 per cent of the sample.
Table 4: The number of regions in each formed club, by jurisdiction
Clubs | Region Total | Share 1/totala | Share 2/totalb | ||||
Jurisdiction | 1 | 2 | 3 | 4 | |||
Australian Capital Territory (ACT) | 1 | 0 | 0 | 1 | 2 | 0.50 | 0.50 |
New South Wales (NSW) | 13 | 10 | 3 | 1 | 27 | 0.48 | 0.85 |
Northern Territory (NT) | 0 | 2 | 0 | 0 | 2 | 0.00 | 1.00 |
Queensland (QLD) | 8 | 6 | 2 | 2 | 18 | 0.44 | 0.79 |
South Australia (SA) | 3 | 2 | 2 | 0 | 7 | 0.43 | 0.71 |
Tasmania (TAS) | 3 | 1 | 0 | 0 | 4 | 0.75 | 1.00 |
Victoria (VIC) | 9 | 8 | 0 | 0 | 17 | 0.53 | 1.00 |
Western Australia (WA) | 5 | 4 | 0 | 1 | 10 | 0.50 | 0.90 |
Total | 42 | 33 | 7 | 5 | 87 | 0.48 | 0.86 |
Notes:
a. Total captures the number of regions in Club 1 to total number of regions in each jurisdiction.
b. Total captures the number of regions in Club 1 and 2 to total number of regions in each jurisdiction.
Also from Table 4, we can see that the second formed club consists of 33 regions out of the 45 remaining, and within this subgroup, there are 10 regions from NSW, eight regions from VIC and six regions from QLD. As members of Club 2 these 33 regions are considered the second most active and energetic regions in terms of EV adoption. The remaining 12 regions in the sample form the third and the fourth convergence clubs. The regions in this are the slowest EV adopters in the sample.
The first ‘Share’ column shows the rate of Club 1 membership in each jurisdiction. It is seen that ACT, TAS, VIC and WA have at least half of their regions belonging to Club 1, the club of fastest adapters. TAS scored the highest rating at 0.75 indicating that three of its four regions have adopted EVs relatively quickly in the last eight years. Furthermore, the fourth TAS region was found to belong to Club 2 – the club of second fastest adaptors – which implies that EV adoption rates in this ‘apple’ state were quite similar between regions and that no region in this state lagged. Meanwhile, it is seen that NSW just missed the 50 per cent cut off for Club 1 membership, with 48 per cent of its regions belonging to Club 1 while the rest identify with others in slower adaptors clubs. NSW sits alongside QLD and SA with ratings that are below 50 per cent which indicates a more uneven level of EV adoption within each jurisdiction1 and increased chances of having lagging regions.
These trends are confirmed with the numbers found in the next ‘Share’ column which shows each state’s membership rate when Clubs 1 and 2 are combined. VIC and TAS lead the pack with all their regions found to belong to this combined club of fastest adaptors. Further, our club convergence analysis showed an absence of regions belonging to Clubs 3 or 4 indicating significant homogeneity in fast EV adoption behaviour within both states. In contrast, our results also showed that while close of half of regions in NSW are fast adaptors, 4 NSW regions or 15 per cent of regions in this most populous state lag in terms of EV adoption. Similarly, lagging regions in QLD and SA comprise 21 and 29 per cent of the state total, respectively.
A visual representation of our club convergence results can be found in the Appendix. As can be seen, regions that comprise Club 1 are found in urban centres of population with high‑density development for commercial and residential types. They include all Australian major cities, except Darwin in NT, as well as highly urbanised neighbouring regions. Our maps also show that regions belonging to Club 3 and Club 4 are located outside the city centres and tend to have low population density levels. These results were consistent with the expectation that the urbanised central regions within each state or territory will tend to lead others in terms of EV adoption. Conversely, they confirm that regions in the more remote locations and with very low population densities rates will tend to lag.
Collectively, our club convergence analysis strongly suggests the existence of lagging regions within Australian states, particularly for NSW, QLD and SA. This shows that EV adoption rates have been far from uniform within each state, and that state-level policies promoting adoption may need to be differentiated between urbanised centres and remote areas to ensure no region is left behind.
Footnotes
[1] We exclude ACT and NT here due to having just 2 regions in each one.
6. Modelling determinants of electric vehicle adoption
In this section, we examine the impact of green awareness on EV adoption rates, alongside a range of other region‑level variables such as income, education and employment rates.
Our empirical strategy involves the estimation of the following basic equation form:

where, for any given region i and time t, EV is the ratio of registered electric vehicles to total vehicle registration, GA is green awareness measured by the number of solar installations per capita, INC is the region’s annual per capita income, EDU is the proportion of population that completed Year 12, FEM is the female-to-male ratio, UNEMP is the unemployment rate and GINI is the level of inequality. We have added these region‑level variables to the models to test whether they are also key factors influencing EV uptake in Australia, as has been found in other economies. Year‑fixed effects are included to eliminate bias from any unobserved variables that evolve over time but are constant across entities. We report our results in Table 5 below. Table 6 contains summary statistics using the modelling exercise in Section 6, and a correlation matrix of the relevant variables to facilitate discussion.
Models 1 and 2 are the models which incorporate all the hypothesised regional variables listed above. Estimation results for Model 1 shows that GA, INC and EDU all impact on EV demand significantly, while FEM, UNEMP and GINI are not significant factors. Model 2 is a modified version of Model 1, in that it includes the same regressors as in Model 1 but also includes dummy variables for the year and is a time‑fixed effects variant. Estimation results for Model 2 show that the impact of GA as a factor in EV is diminished with the addition of year dummies, but INC and EDU remain significant influences. FEM, UNEMP and GINI remain insignificant factors, as in Model 1.
Experienced analysts may observe that there is a high chance of multicollinearity within these models, which, if true, would result in less reliable coefficient estimates for β2 and β3. Multicollinearity can lead to skewed or misleading results as the high correlation between INC and EDU, for example, makes it difficult to separate individual effects on EV demand in a statistical model. Technically, multicollinearity can lead to wider confidence intervals and produce less reliable probabilities in terms of the independent variable effects. A quick inspection of the correlation matrix of the variables in the models (Table 6) reveals that INC and EDU have a strong linear relationship (ρ = 0.768). Given this, we proceed to estimate Models 3–6 by using only INC in the model (we drop EDU), along with the other variables. And as a matter of procedure, we also estimate Models 7–10 using only EDU in the model (we drop INC), along with the other variables. The results are presented in the rests of the columns in Table 5.
Model 3 shows that GA, INC and GINI are the factors that exert a significant influence on EV demand, while FEM and UNEMP are not significant. Of the significant variables, we can further say that a 10 per cent increase green awareness leads to a 2.1 per cent increase in EV registrations, all other variables remaining constant. For INC, the results indicate that a 10 per cent increase in per capita incomes is associated with an increase in EV adoption rate of about 27 per cent. For GINI, the significant negative coefficients indicates that a 10 per cent decrease in a region’s inequality level raises EV demand by 12.82 per cent or 13 per cent rounded up. Model 4 results were obtained by incorporating time-year effects in the estimation. Under this, we can see that the impact of GA on EV demand becomes insignificant, but INC and GINI remain significant factors of similar magnitudes to Model 3.
In Models 5 and 6, we continue to use INC on the right-hand side, at the expense of EDU. We also exclude UNEMP to eliminate any multicollinearity effects between INC and UNEMP. Table 6 shows that INC and UMEMP has a ρ = 0.611. Model 5 results show the significance of GA, INC and GINI, and with a similar size and impact as in Model 3. Time‑fixed effects Model 6 return results that show no significance for GA, but INC, FEM and GINI all return as highly significant factors. The size impact of INC and GINI on EV demand are similar to those we have already seen in Models 3-5. The new result here demonstrates the significance of FEM. Assuming all else constant, Model 6 reveals that an increase in the proportion of females relative to males in the region (FEM) increases EV demand by 24.34 per cent.
Table 5. Final Regression Results
VARIABLES | (1) | (2) | (3) | (4) | (5) | (6) | (7) | (8) | (9) | (10) |
---|---|---|---|---|---|---|---|---|---|---|
Log GA | 0.205*** | -0.038 | 0.210*** | 0.019 | 0.208*** | 0.017 | 0.221*** | -0.053 | 0.160*** | -0.080 |
(0.054) | (0.054) | (0.054) | (0.055) | (0.054) | (0.055) | (0.055) | (0.054) | (0.051) | (0.049) | |
Log INC | 1.968*** | 0.791*** | 2.710*** | 2.309*** | 2.655*** | 2.369*** | ||||
(0.309) | (0.306) | (0.230) | (0.222) | (0.181) | (0.174) | |||||
Log EDU | 0.856*** | 1.624*** | 1.888*** | 2.054*** | 1.971*** | 2.087*** | ||||
(0.240) | (0.233) | (0.183) | (0.164) | (0.181) | (0.162) | |||||
Log POP | 0.129 | 0.803 | 1.258 | 2.605** | 1.346 | 2.434** | -0.778 | 0.536 | 0.586 | 1.064 |
(1.137) | (1.045) | (1.101) | (1.047) | (1.051) | (0.997) | (1.160) | (1.044) | (1.067) | (0.951) | |
Log EMP | 0.092 | -0.002 | 0.054 | -0.050 | -0.237* | -0.123 | -0.294** | -0.142 | ||
(0.129) | (0.119) | (0.130) | (0.123) | (0.122) | (0.110) | (0.121) | (0.109) | |||
Log GINI | -0.664 | -0.224 | -1.282** | -1.387** | -1.234** | -1.404** | 1.537*** | 0.586 | ||
(0.619) | (0.569) | (0.599) | (0.563) | (0.593) | (0.558) | (0.527) | (0.478) | |||
Constant | -34.859*** | -23.620*** | -39.680*** | -34.271*** | -38.876*** | -35.061*** | -15.663*** | -15.927*** | -16.519*** | -16.248*** |
(3.191) | (3.123) | (2.915) | (2.818) | (2.152) | (2.085) | (1.072) | (0.964) | (1.037) | (0.928) | |
Fixed Effects | n | y | n | y | n | y | n | y | n | y |
Observations | 695 | 695 | 695 | 695 | 696 | 696 | 695 | 695 | 695 | 695 |
R-squared | 0.310 | 0.427 | 0.297 | 0.386 | 0.298 | 0.386 | 0.270 | 0.421 | 0.261 | 0.420 |
Standard errors in parentheses *** p<0.01, ** p<0.05, * p<0.1 |
Note: Standard errors in parentheses *** p<0.01, ** p<0.05, * p<0.1
Table 6. Summary Statistics (of variables used in Section 6 models)
EV | GA | INC | EDU | FEM | UNEMP | GINI | |
---|---|---|---|---|---|---|---|
Mean | 0.00034 | 0.011 | 34114 | 48 | 1.012 | 3.1 | 0.465 |
Median | 0.00017 | 0.010 | 31220 | 46 | 1.018 | 3.2 | 0.459 |
Max | 0.00803 | 0.029 | 73754 | 76 | 1.070 | 6.2 | 0.610 |
Min | 0.00001 | 0.001 | 19089 | 27 | 0.760 | 2.8 | 0.389 |
Std Dev | 0.00064 | 0.006 | 9798 | 13 | 0.040 | 3.2 | 0.042 |
Correlation matrix | |||||||
EV | 1.000 | ||||||
GA | -0.152 | 1.000 | |||||
INC | 0.511 | -0.358 | 1.000 | ||||
EDU | 0.495 | -0.473 | 0.768 | 1.000 | |||
FEM | 0.123 | 0.148 | 0.090 | 0.206 | 1.000 | ||
UNEMP | -0.286 | 0.311 | -0.611 | -0.480 | 0.251 | 1.000 | |
GINI | 0.220 | -0.251 | 0.578 | 0.323 | 0.294 | -0.208 | 1.000 |
In Models 7–10, we use the EDU variable instead of the INC variable as a key regressor in the model. We find in Model 7 that GA, EDU and GINI are all significant factors on EV demand but note that the sign of the GINI coefficient is positive, unlike in previous models. A positive coefficient for the GINI is not as expected and is probably harder to rationalise compared to the negative coefficient found earlier. We also see in this model that UNEMP is negatively significant, albeit at the 10 per cent level only. This means that a 10 per cent decline in unemployment rates in the region can result in a 2 per cent increase in EV registrations, which is plausible. The next model Model 8, which is the time‑fixed effects version of Model 7, returns with weak results – none of the coefficients returned significant except for the coefficient of EDU. This model appears less reliable and less informative than the others we have already reviewed.
Lastly, we consider Models 9 and 10 found in the last two columns of Table 5. In Model 9, we find that GA and EDU are strongly significant factors that influence EV demand positively. In terms of size, the effect of GA appears relatively small compared to earlier models. The size impact of EDU appears close in value compared to those found in Models 7 and 8. We also see in this model that UNEMP is negatively highly significant. The coefficient value of -0.294 means that a 10 per cent decline in a regional unemployment rate results in a 2 per cent increase in EV registrations, which is also plausible. Model 10 results appear weaker compared to that of Model 9. With only one regressor showing significance, this model appears less reliable and less informative than the others already considered.
Models 5 and 6 present the most plausible model of all: green awareness, income, female population and inequality are found to influence EV adoption rates across the board. In Model 5, we find that a 10 per cent increase in green awareness results in a 2 per cent increase in EV registrations, all else being constant. Similarly, a 10 per cent increase in per capita income results in a significant increase in EV registrations of around 24–26 per cent. A 10 per cent decrease in inequality levels within a region is also found to raise EV demand by between 12–14 per cent. Finally, a 10 per cent increase in the number of females in the region relative to males raises EV demand by about 24 per cent.
7. Conclusion
The study examined the path of 87 regions of Australian towards adoption of electric vehicles using club convergence analysis.
The results show no overall convergence in EV adoption across Australia's regions. Instead, different steady‑state equilibria exist with the algorithm detecting convergence to four clusters.
Club convergence analysis revealed that Australian regions vary widely in terms of their green awareness, and that there is a clear separation between the peripheral and higher-density regions at the heart of the states or territories. For regions in city centres, the likelihood of clustering at the top group of adopters remains higher than for regions outside the city centres. A key result found that while some regions in a particular jurisdiction appeared in the higher club of adoption, others in the same jurisdiction were found to belong to the lowest club of electric vehicle adopters. This spread of membership across the clubs suggests a wide gap in adoption rates across areas within the same state or territory.
These findings provide new data insights of particular importance to Victoria. Across the four most populous states, VIC leads the pack in terms of regional homogeneity in EV take up rates. We found 100 per cent of regions in VIC are fast EV adopters which is a rate that is far above those found for other key states in the country: NSW (85 per cent), QLD (79 per cent) and WA (90 per cent) (see Table 4). This implies that the issue of lagging regions in the sense of EV adoption is relatively minimized in VIC. These results are consistent with those indicated in the VFACTS 2023 report of the Federation Chamber of Automotive Industries (FCAI) and provide strong empirical support to the claim that VIC leads in the rate of decarbonisation in the country1.
Our econometric modelling of macroeconomic determinants of electric vehicle adoption further revealed that a region’s green awareness, per capita income, educational attainment and level of inequality are the strongest region-level factors influencing EV adoption. Our findings are consistent with those found in other countries, but this study is the first to confirm these results for Australia.
In conclusion, this study explored the observed variations in regional adoption rates to understand trends and patterns of development, and how these can affect EV adoption behaviour over the foreseeable future. It provided new data insights that can be used to support strategic action for accelerating the adoption of EV technology across all regions of Australia.
Footnotes
[1] Premier of Victoria, Setting An Ambitious Emissions Reduction Target, accessed 3 Aug 2023.
8. References
References for this Victoria’s Economic Bulletin research article.
Abotalebi, E., Scott, D. M. & Ferguson, M. R. Why is electric vehicle uptake low in Atlantic Canada? A comparison to leading adoption provinces. Journal of Transport Geography, 2019, 74: 289-298.
Anastasiadou, K and Gavanas, N. State-of-the-Art Review of the Key Factors Affecting Electric Vehicle Adoption by Consumers. Energies, 2022, 15: 9409.
Australia Institute, Climate of the Nation Report 2022(opens in a new window)
Awaworyi Churchill, S., Inekwe, J. & Ivanovski, K. Conditional convergence in per capita carbon emissions since 1900. Applied Energy, 2018, 228: 916-927.
Beresteanu, A., & Li, S. Gasoline prices, government support, and the demand for hybrid vehicles in the United States. International Economic Review, 2011, 52(1): 161-182.
Bhattacharya, M., & Inekwe, J. Convergence in sovereign debt defaults: quantifying the roles of institutions. Oxford Bulletin of Economics and Statistics, 2021, 83(3) 792-811.
Bhattacharya, M., Inekwe, J. N. & Sadorsky, P. Consumption-based and territory‑based carbon emissions intensity: Determinants and forecasting using club convergence across countries. Energy Economics, 2020a, 86: 104632.
Bhattacharya, M., Inekwe, J. N.& Sadorsky, P. Convergence of energy productivity in Australian states and territories: Determinants and forecasts. Energy Economics, (2020b), 85:104538.
Bhattacharya, M., Inekwe, J. N., Sadorsky, P. & Saha, A. Convergence of energy productivity across Indian states and territories. Energy Economics, 2018, 74: 427‑440.
Biresselioglu, M. E., Kaplan, M. D. & Yilmaz, B. K.. Electric mobility in Europe: A comprehensive review of motivators and barriers in decision making processes. Transportation Research Part A: Policy and Practice, 2018, 109: 1-13.
Buhmann, K. M., & Criado, J. R.. Consumers' preferences for electric vehicles: the role of status and reputation. Transportation research part D: transport and environment, 2023, 114: 103530.
Clean Energy Council Australia (2021). Clean Energy Australia Report 2021(opens in a new window) (PDF). Clean Energy Australia. Accessed 17 July 2023.
Diamond, D. The impact of government incentives for hybrid-electric vehicles: Evidence from US states. Energy policy, 2009, 37(3): 972-983.
Electric Vehicle Council Annual Reports (2022, 2023).
Electric Vehicle Council Consumer Attitudes Survey 2021(opens in a new window), Australia, 2021.
Ferguson, M., Mohamed, M., Higgins, C.D., Abotalebi, E., Kanaroglou, P. How open are Canadian households to electric vehicles? A national latent class choice analysis with willingness-to-pay and metropolitan characterization. Transp. Res. Part D: Transp. Environ. 2018, 58: 208–224.
Hardman, S., Chandan, A., Tal, G. & Turrentine, T. The effectiveness of financial purchase incentives for battery electric vehicles - A review of the evidence. Renewable and Sustainable Energy Reviews, 2017, 80: 1100-1111.
Hidrue, M. K., Parsons, G. R., Kempton, W. & Gardner, M. P. Willingness to pay for electric vehicles and their attributes. Resource and energy economics, 2011, 33(3): 686-705.
Hodrick RJ, Prescott EC. Postwar US business cycles: an empirical investigation. Journal of Money, Credit and Banking, 1997, 29:1–16
International Energy Agency The Role of Critical Minerals in Clean Energy Transitions IEA website, accessed 10 February 2023.
Li, L., Wang, Z. & Xie, X. From government to market? A discrete choice analysis of policy instruments for electric vehicle adoption. Transportation Research Part A: Policy and Practice, 2022, 160: 143-159.
Li, L., Wang, Z., Gong, Y. & Liu, S.. Self-image motives for electric vehicle adoption: Evidence from China. Transportation Research Part D: Transport and Environment, 2022, 109: 103383.
Mau, P., Eyzaguirre, J., Jaccard, M., Collins-Dodd, C., & Tiedemann, K.. The ‘neighbor effect’: Simulating dynamics in consumer preferences for new vehicle technologies. Ecological Economics, 2008, 68(1-2): 504-516.
Mersky, A. C., Sprei, F., Samaras, C., & Qian, Z. S.. Effectiveness of incentives on electric vehicle adoption in Norway. Transportation Research Part D: Transport and Environment, 2016, 46: 56-68.
Mohamed, M., Higgins, C., Ferguson, M., Kanaroglou, P. Identifying and characterizing potential electric vehicle adopters in Canada: a two-stage modelling approach. Transport Policy, 2016, 52: 100–112.
Mustafa, S., Zhang, W., & Li, R. Does environmental awareness play a role in EV adoption? A value-based adoption model analysis with SEM-ANN approach. IEEE/WIC/ACM International Conference on Web Intelligence and Intelligent Agent Technology, 2021, 433-440.
Phillips, P. C. B., & Sul, D. Transition modeling and econometric convergence tests. Econometrica, 2007, 75(6): 1771-1855. doi:DOI 10.1111/j.1468-0262.2007.00811.
Phillips, P. C. B., & Sul, D. Economic Transition and Growth. Journal of Applied Econometrics, 2009, 24(7): 1153-1185.
Okada, T., Tamaki, T., & Managi, S. Effect of environmental awareness on purchase intention and satisfaction pertaining to electric vehicles in Japan. Transportation Research Part D: Transport and Environment, 2019, 67: 503-513.
Potoglou, D. & Kanaroglou, P. S. Household demand and willingness to pay for clean vehicles. Transportation Research Part D: Transport and Environment, 2007, 12(4): 264-274.
Purtill, J. Australians want to buy electric cars, but car makers say government policy blocks supply(opens in a new window). Australian Broadcasting Corporation, April 2021
Ritter, Á. M. Borchardt, M., Vaccaro, G. L., Pereira, G. M., & Almeida, F.. Motivations for promoting the consumption of green products in an emerging country: exploring attitudes of Brazilian consumers. Journal of Cleaner Production, 2015, 106: 507-520.
Salisbury, M. & Toor, W. How and why leading utilities are embracing electric vehicles. The Electricity Journal, 2016, 29(6): 22-27.
Xiong, R., Pan, Y., Shen, W., Li, H. & Sun, F. Lithium-ion battery aging mechanisms and diagnosis method for automotive applications: Recent advances and perspectives. Renewable and Sustainable Energy Reviews, 2020, 131: 110048.
Zhang, G., Wei, X., Tang, X., Zhu, J., Chen, S., & Dai, H. Internal short circuit mechanisms, experimental approaches and detection methods of lithium-ion batteries for electric vehicles: A review. Renewable and Sustainable Energy Reviews, 2021,141: 110790.
Zhang, Y., Yu, Y., & Zou, B. Analyzing public awareness and acceptance of alternative fuel vehicles in China: The case of EV. Energy Policy, 2011, 39(11): 7015-7024.
9. Appendix
Club convergence maps by states and territories.
Figure 1: Club composition in New South Wales
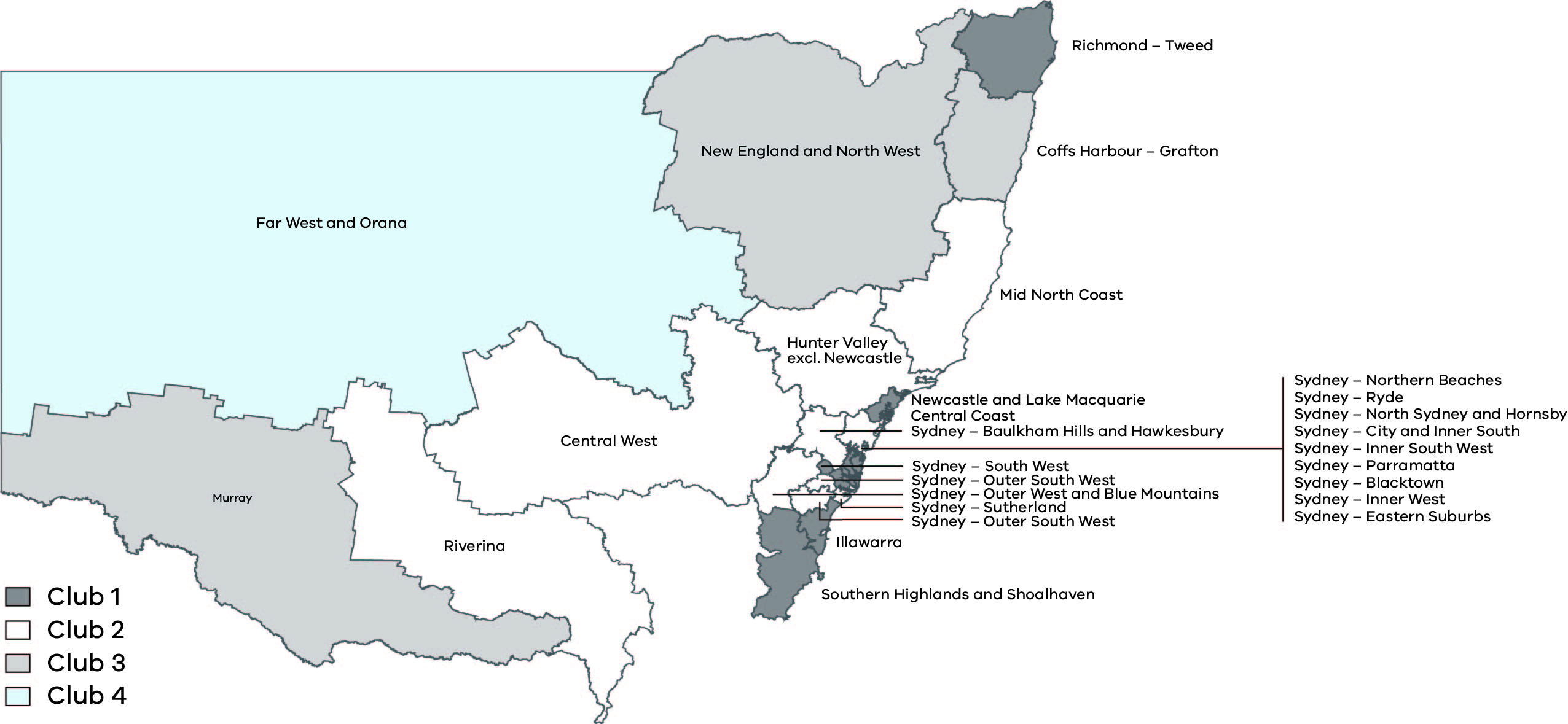
Figure 2: Club composition in Victoria
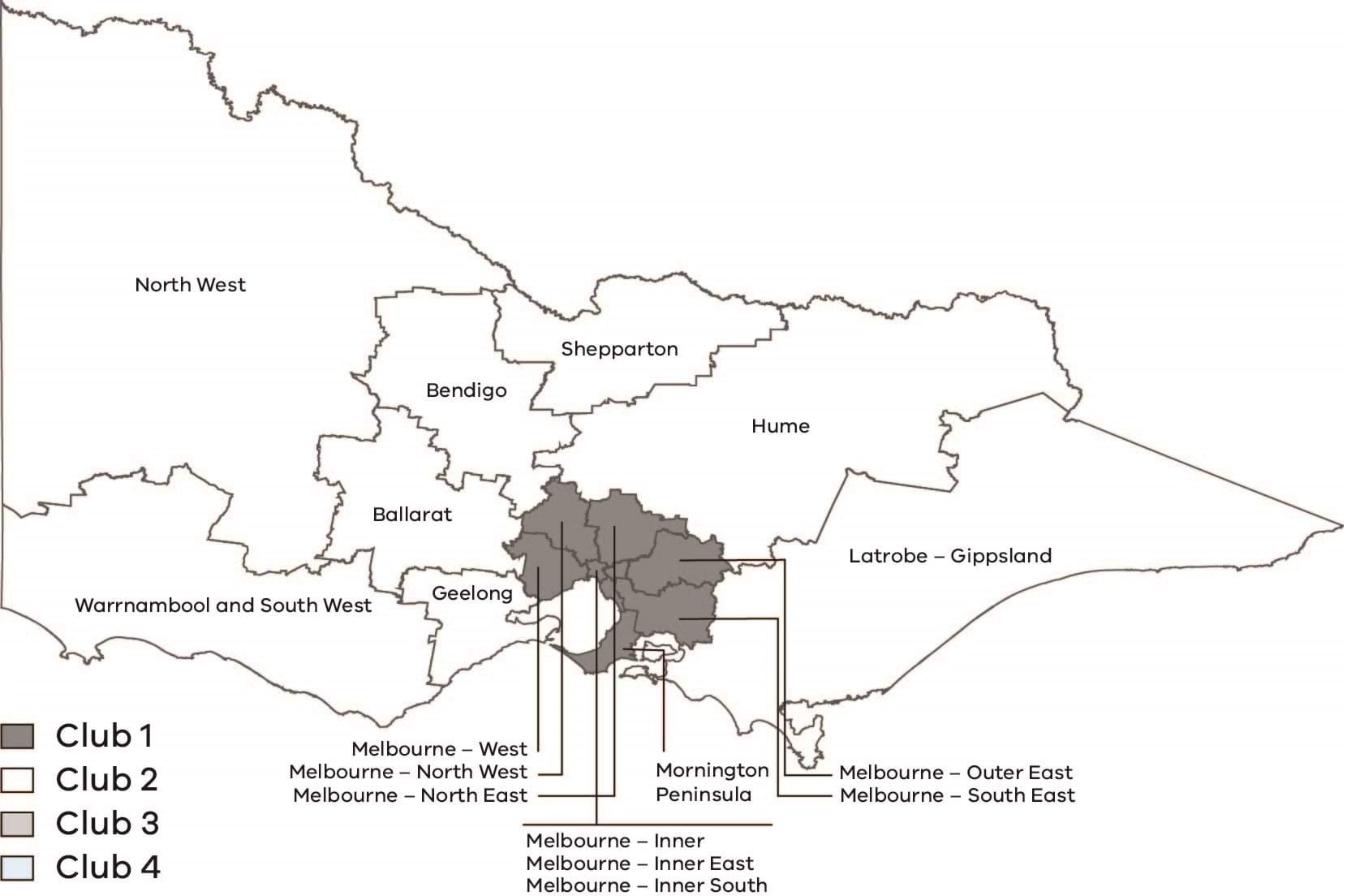
Figure 3: Club composition in Queensland and the Northern Territory
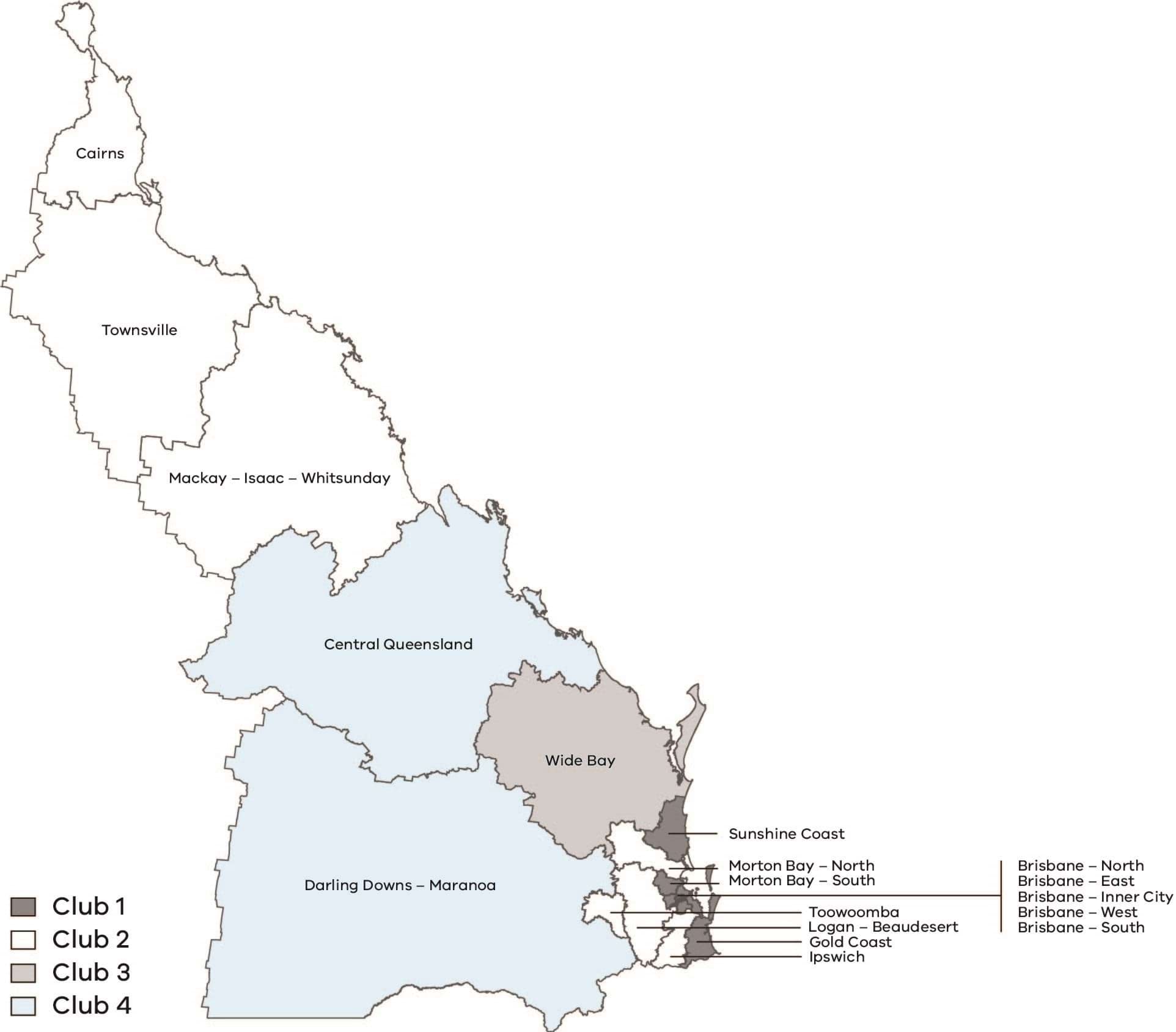
Note: In this study, SA4level data required were not available from ABS due confidentiality restrictions from very low population counts. The maps above for Queensland and the Northern Territory capture those SA4 areas which we were able to use in the analysis. For more detailed information on these data limitations, please see the ABS website.
Figure 4: Club composition in South Australia
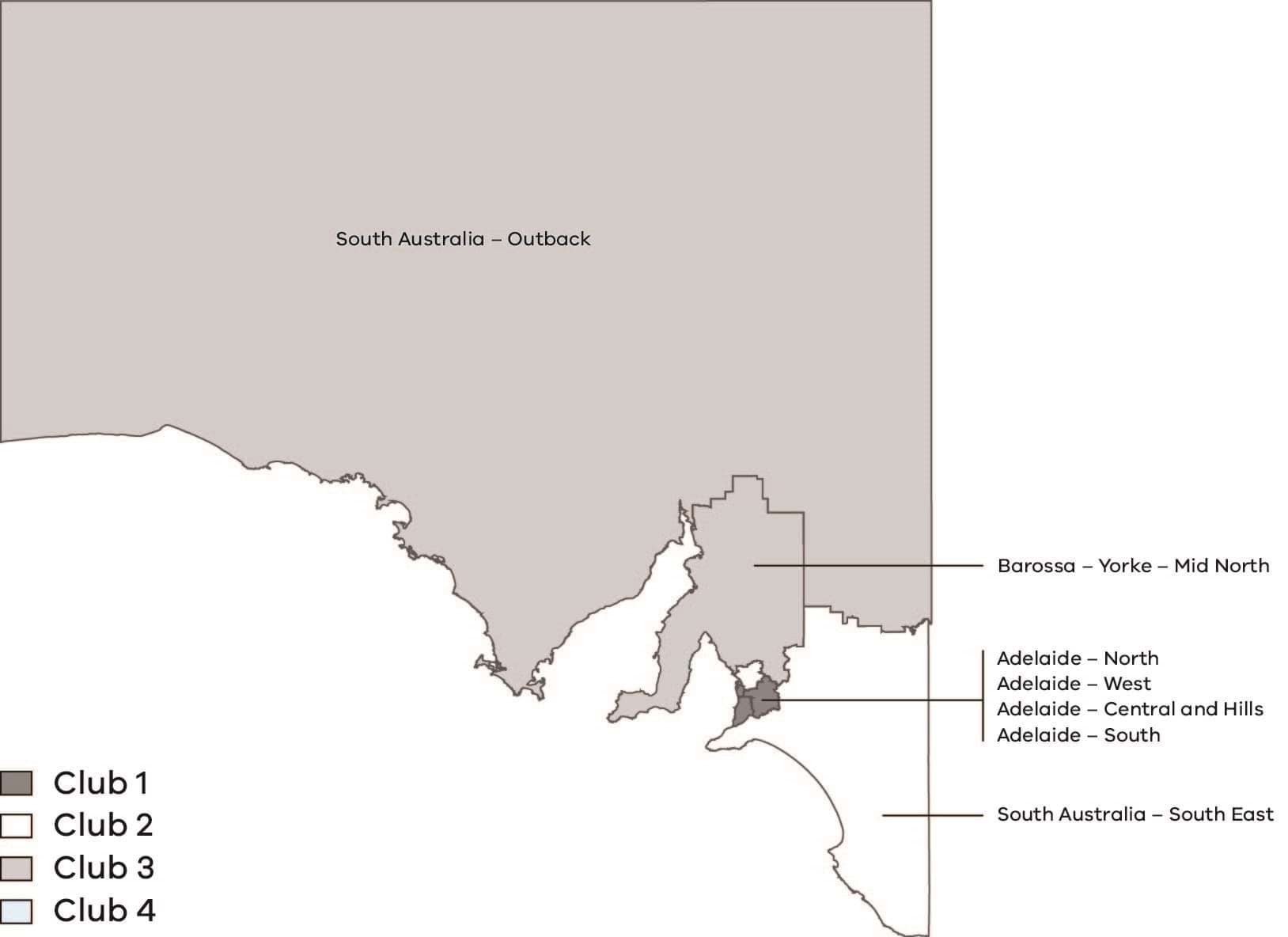
Figure 5: Club composition in Western Australia
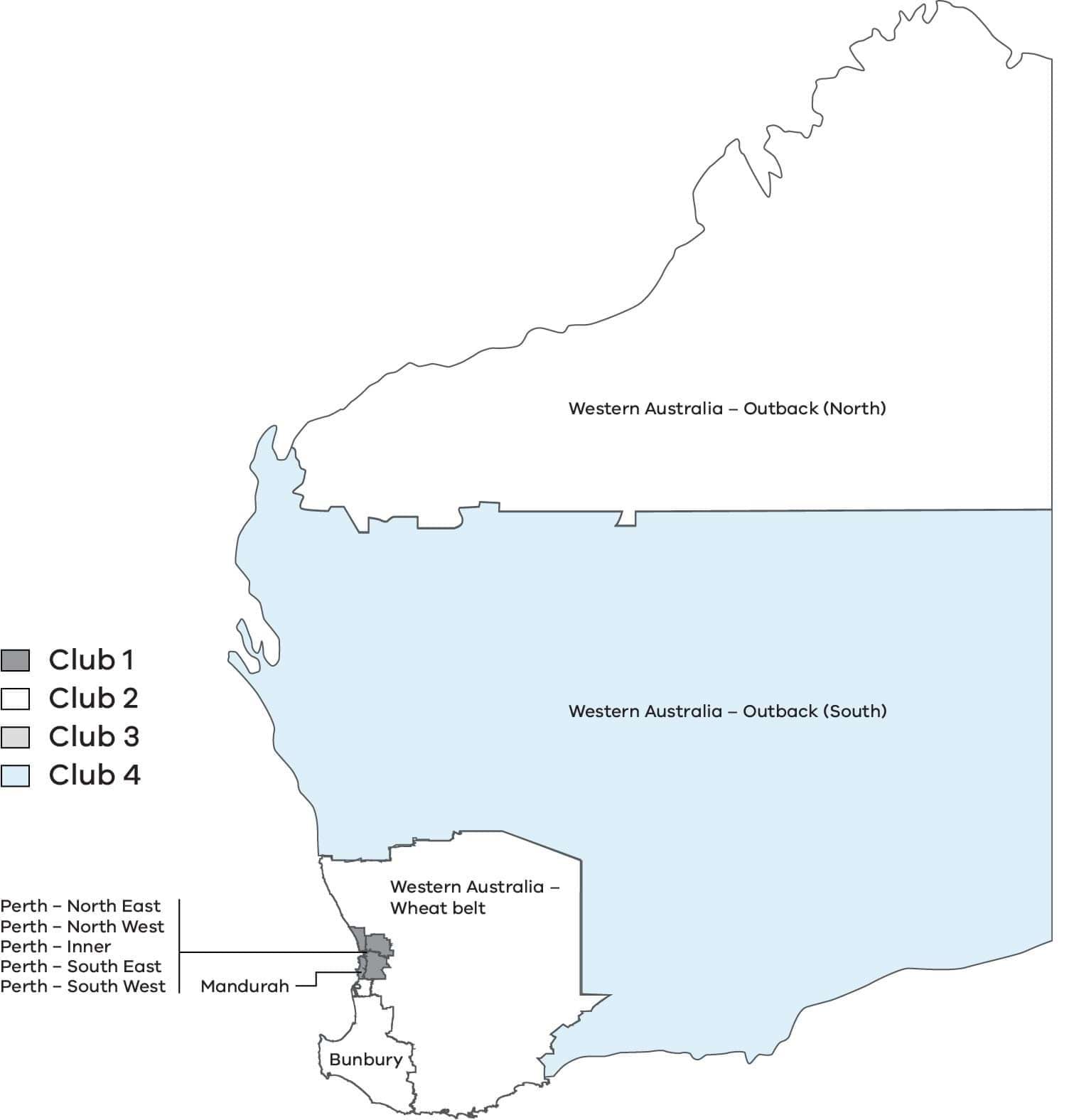
Figure 6: Club composition in Tasmania
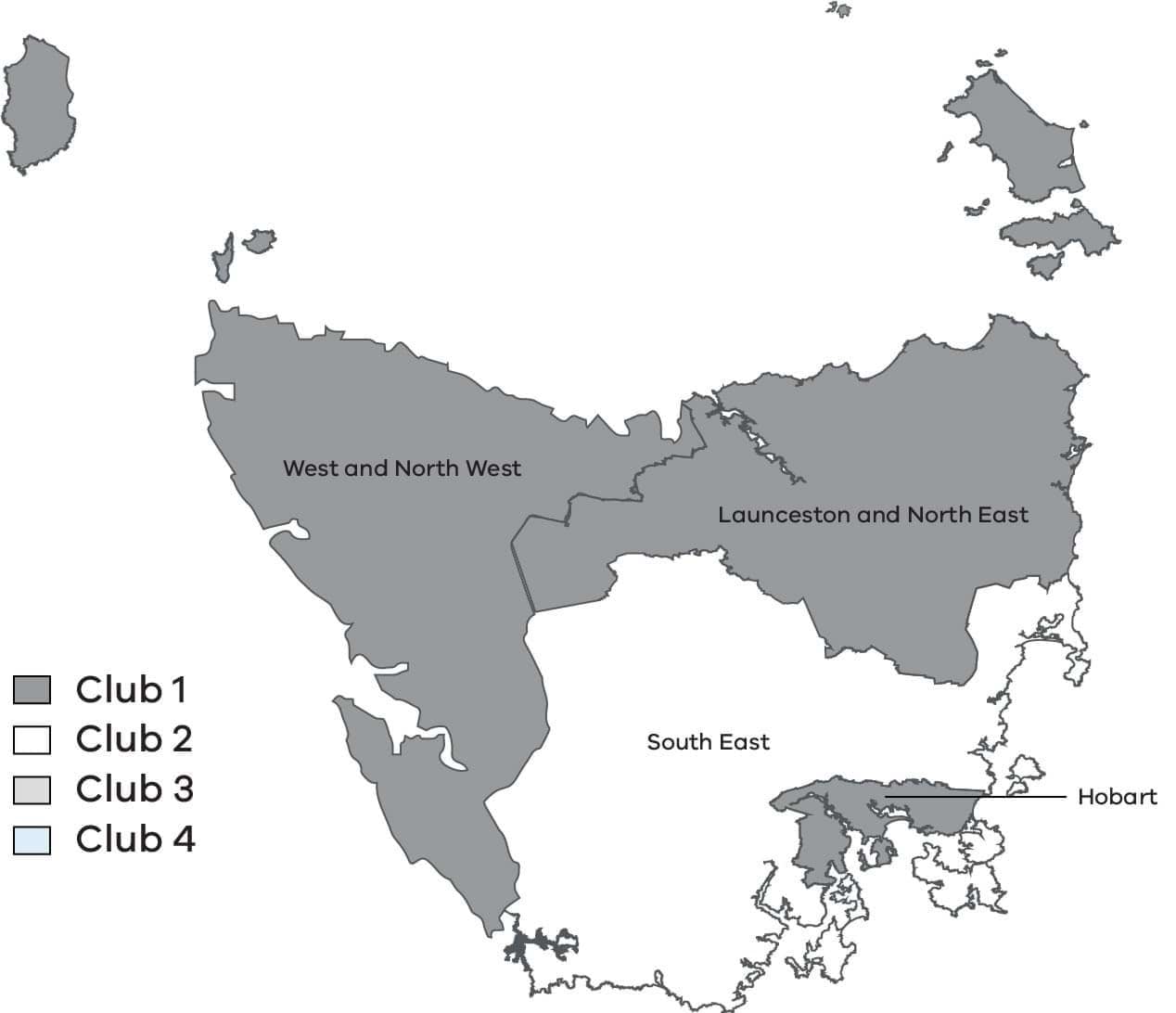